In this article by Giorgio Sarno, Senior Data Scientist, Stratio Automotive, we explore how Artificial Intelligence and Machine Learning systems can make public transport a greener and more reliable service, and a more obvious choice for commuters.
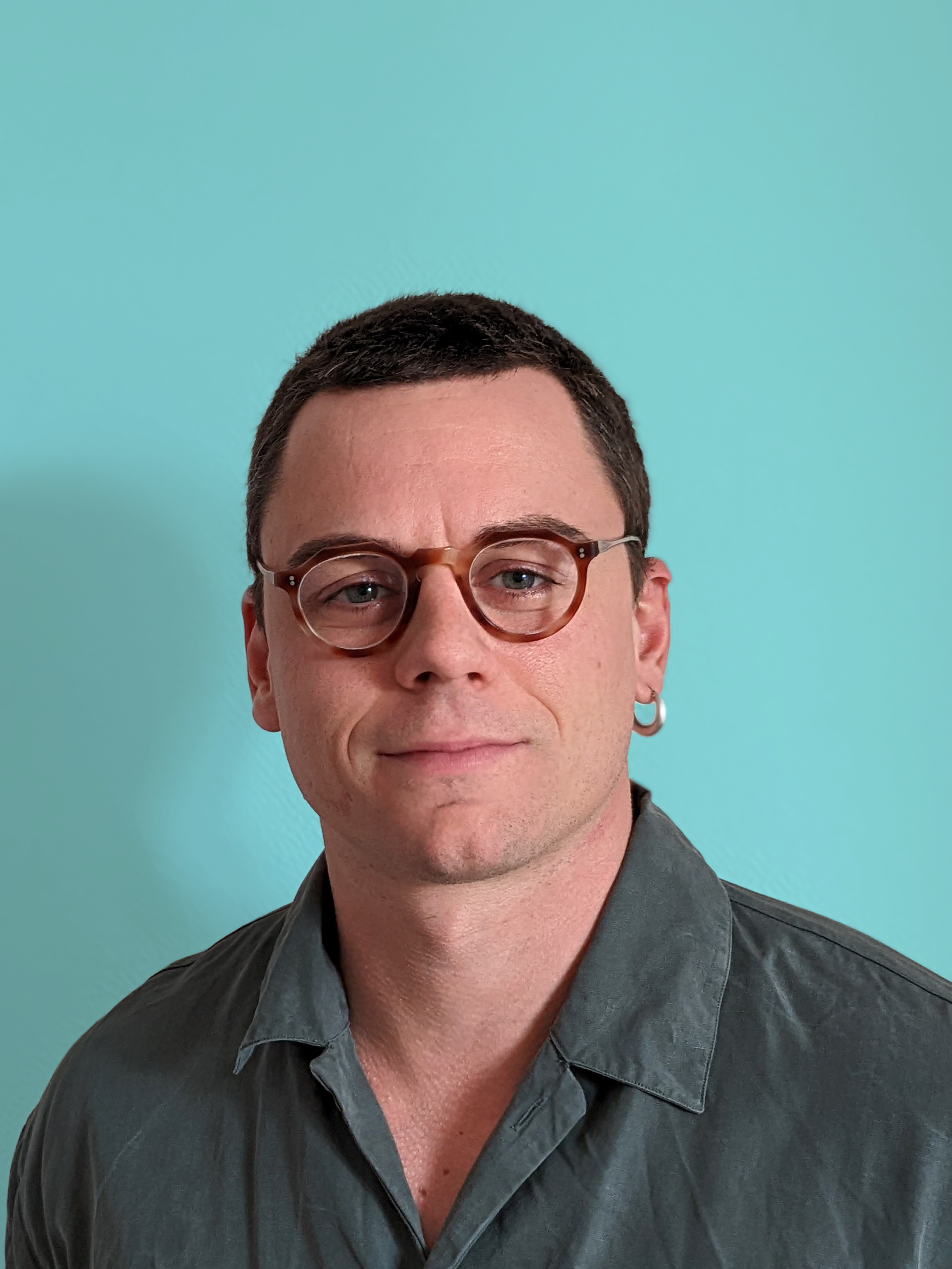
Public transport has long been cited as a ‘greener’ way of travelling but unfortunately, due to the historical unreliability of the bus services across the country, many people in Britain still heavily rely on cars to get about. Since the up-front cost of transitioning to electric vehicles (EVs) remains high (around £50,000), most people still drive petrol and diesel vehicles, with lots of households even having more than one of these types of cars.
The difficulties of transitioning to EVs have been recently acknowledged by Prime Minister, Rishi Sunak, who made the decision to delay the ban on sale of new petrol and diesel cars and push back Britain’s net zero goals to reduce living cost pressures. This doesn’t paint a very pretty picture for reducing emissions in our urban areas but reinforces the view that the onus should be on transport companies to lead the charge when it comes to making green transport more accessible.
Public transport providers’ hesitancy to invest in EVs comes from a lack of understanding about the tools and knowledge they need to make the switch profitable – as well as the significant upfront investment of course, which is even higher for fleet operators than it is for the consumer. Artificial Intelligence (AI) and Machine Learning (ML) are going to be key in supporting them to become greener. These technologies can provide greater insights and data analysis to improve reliability and operate both new EV and older internal combustion engine (ICE) vehicles more efficiently and cost-effectively.
Easing the transition to EV Fleets
The first thing AI and ML technology can do for bus operators is to ease and expedite the costly transition to EVs. The single biggest concern with regards to EV transition is the upfront investment, which is high on account of the battery packs. In fact, this component makes up around 40% of the total cost of an electric bus.
AI and ML technology is now offering practical solutions to maintain and optimise EV battery life for enhanced route planning, minimal disruptions and lower maintenance costs. By looking at State of Charge (SoC) and Depth of Discharge (DoC) data, fleet managers can identify where the most effective charging points are, predict energy usage in variable conditions (such as severe weather or heavy traffic) and ultimately limit the degradation of the battery.
Leveraging this technology offers operators a 360-degree view of their EV transition, and can give them more confidence that they will get back the initial investment, on top of delivering a more reliable service.
AI-powered eco-driving strategies
The second key benefit that transport operators can access with AI and ML relates to ongoing operational strategy. Using AI and ML to create eco-driving/fuel-efficiency strategies in internal combustion engine vehicles saves fuel and therefore cuts carbon emissions. In fact, the digitisation of vehicles enables the implementation of behaviour-based Eco driving strategies that can inform fleet managers and drivers on how to optimise operations and minimise consumption.
Driver behaviour data and information on idle time performance can be ingested by AI and ML models to generate actionable insights on how a fleet can reduce its overall carbon emissions. This has already been tried-and-tested by Arriva, which has saved 942 litres of diesel per vehicle per year in the Czech Republic, equating to 2.6 tonnes of carbon dioxide emissions avoided per vehicle, per year.
Not only this but leveraging AI and ML for predictive maintenance reduces unexpected breakdowns and optimises the replacement of components. This limits waste generation and reduces the need for new equipment production, as parts are only changed when necessary, rather than at pre-set intervals, when they may still have some useful life. This results in a lower environmental impact associated with resource extraction, manufacturing, and disposal.
Improving Reliability
Ultimately, all the benefits of integrating AI and ML models into the operation of both ICE vehicles and new EV fleets lead operators towards increasing the reliability of their service. The data produced and analysed by these models can turn buses from a ‘black box’ to a source of real-time intelligence useful to guarantee a transport network that passengers can trust.
By taking a data-centric approach, bus operators can rely on the continuous improvement of AI algorithms, which increase in accuracy as they ingest more data. As a result, as operators manage to reduce the number of breakdowns, they can offer a genuine reliable alternative to private transport.
With some operators already implementing predictive maintenance, the approach is becoming more ubiquitous. It represents the new frontier when it comes to maximising vehicle availability and ensuring that buses stay on the road, where they need to be, serving passengers.
Making public transport the obvious choice
The relationship between public transport operators and passengers has become increasingly fraught over the years as the fleet managers have failed to keep up with growing customer expectations.
We now live in a world where most of us work from home at least some of the time, do our shopping online and even attend things like doctors appointments and university lectures entirely remotely. This makes even more important to make transportation convenient and practical.
Now is the time for fleet managers to realise the potential of AI and ML technology to entice customers away from private vehicles and onto buses – ultimately cutting back on the number of cars on the road.
Click below to share this article